Navigating the realm of data science unveils an intricate landscape where statistical methodologies, machine learning techniques, and comprehensive data analysis converge to distill vast data oceans into discernible, actionable intelligence. This interdisciplinary domain leverages robust datasets to forecast trends, inform strategic decisions, and tackle complex issues across myriad sectors. Analyzing data patterns and relationships, data science initiatives catalyze innovation and operational efficiency, establishing data science as a crucial pillar in the contemporary, data-centric era. This narrative aims to demystify the core methodologies underpinning data science, accentuating data’s pivotal role in informing our perspectives and catalyzing future breakthroughs.
Gathering Data: Laying the Groundwork for Insight
The data science voyage commences with meticulous data gathering, a critical endeavor that influences the caliber and relevance of derived insights. This initial step involves pinpointing pertinent data points and deploying mechanisms to amass data efficiently, whether through automated processes, observational studies, or digital engagements. The integrity of data science projects hinges on this initial data quality, emphasizing the necessity for comprehensive data gathering strategies. This stage lays the foundational bedrock for all ensuing analysis, ensuring a robust dataset that faithfully represents the studied phenomena.
Data Refinement and Setup: Priming Data for Exploration
Subsequent to data accumulation, attention shifts towards data refinement and setup, a detailed phase that transforms raw data into an analytically amenable format. This phase tackles data discrepancies, errors, and absent values, employing various techniques to normalize and standardize datasets. The precision involved in data refinement not only bolsters the accuracy of data science initiatives but also streamlines the exploration phase, priming the data for effective scrutiny. The meticulous care in refining and setting up data underscores its critical importance in the success trajectory of data science projects.
Scrutinizing Data: Extracting Patterns and Insights
The essence of data science is encapsulated in the data scrutiny phase, where analytical models and statistical methodologies are applied to dissect and comprehend data. Implementing statistical analyses, exploratory investigations, and predictive modeling, data scientists navigate through datasets to unearth significant patterns and insights. This stage is pivotal for decoding the narratives woven within data, laying the groundwork for actionable intelligence and strategic guidance.
Illustrating Data Narratives: Visualizing Insights
Data illustration, both an art and a methodology, empowers data scientists to present complex datasets in intuitive, graphical formats, illuminating key findings and trends. By converting intricate data into accessible visual formats, data illustration bridges the gap between data science and decision-making entities, ensuring insights are comprehensible and actionable. This phase plays a vital role in democratizing data insights, allowing a wider audience to engage with and act upon the presented intelligence.
Predictive Learning: Anticipating the Future
Machine learning, a fundamental pillar of contemporary data science, equips computers with the ability to learn from data, recognizing patterns to make informed forecasts about future occurrences. This involves algorithmic training on historical data, empowering these algorithms to project future trends. Spanning from predicting consumer behaviors to identifying data network anomalies, machine learning showcases its versatility and potency. This technique enhances the efficiency of insight extraction, offering scalable and sophisticated solutions for intricate challenges.
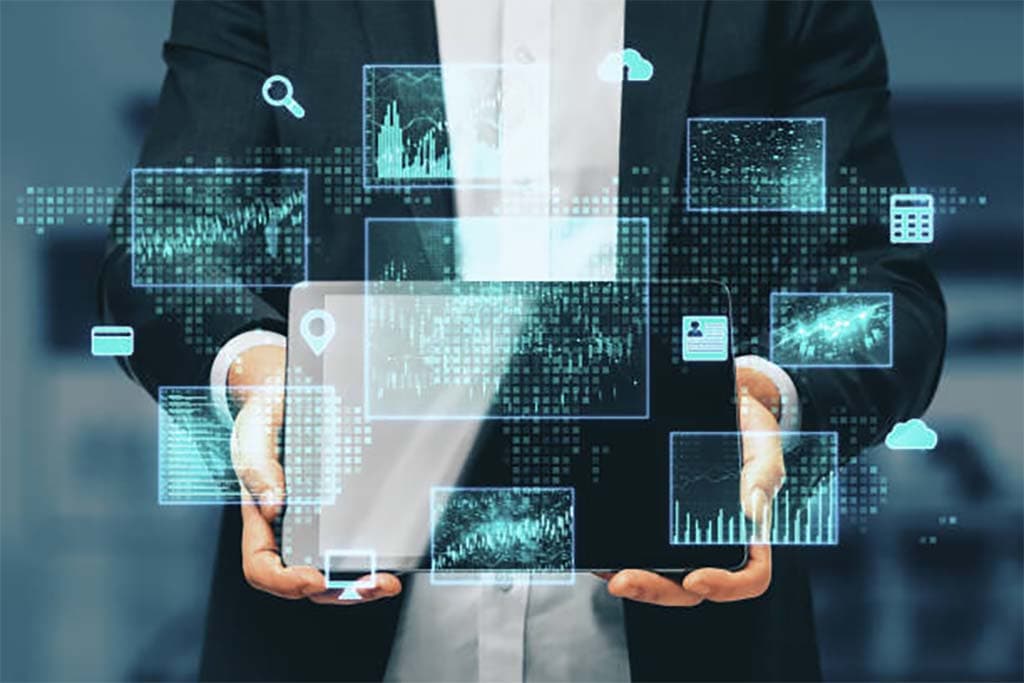
Advanced Predictive Learning: Deep Learning Innovations
Deep learning, a nuanced branch of machine learning, employs layered neural networks to analyze and comprehend complex data patterns. These advanced algorithms, inspired by the human brain’s neural architecture, process data in sequential layers, enabling significant advancements in areas like image and speech recognition. Deep learning’s proficiency in managing diverse and voluminous datasets is transforming data science’s capabilities, elevating the precision and depth of insights derived.
Reflecting on Data Science’s Horizon
As we progress, the significance of data science in mining the burgeoning data reserves will increasingly come to the fore. The methodologies delineated here stand at the vanguard of the field, facilitating the distillation of valuable insights from the growing complexity of global data landscapes. The prospect of data science beckons even greater innovations, with ongoing advancements in analytical tools and techniques. Remaining abreast of these developments, data scientists are poised to play a central role in steering progress, shaping policies, and pioneering new directions in our data-driven society. This examination of data science methodologies is not merely academic; it’s an expedition into the core of contemporary analytical and decision-making processes, energized by the power of data.